Bingliang Zhang
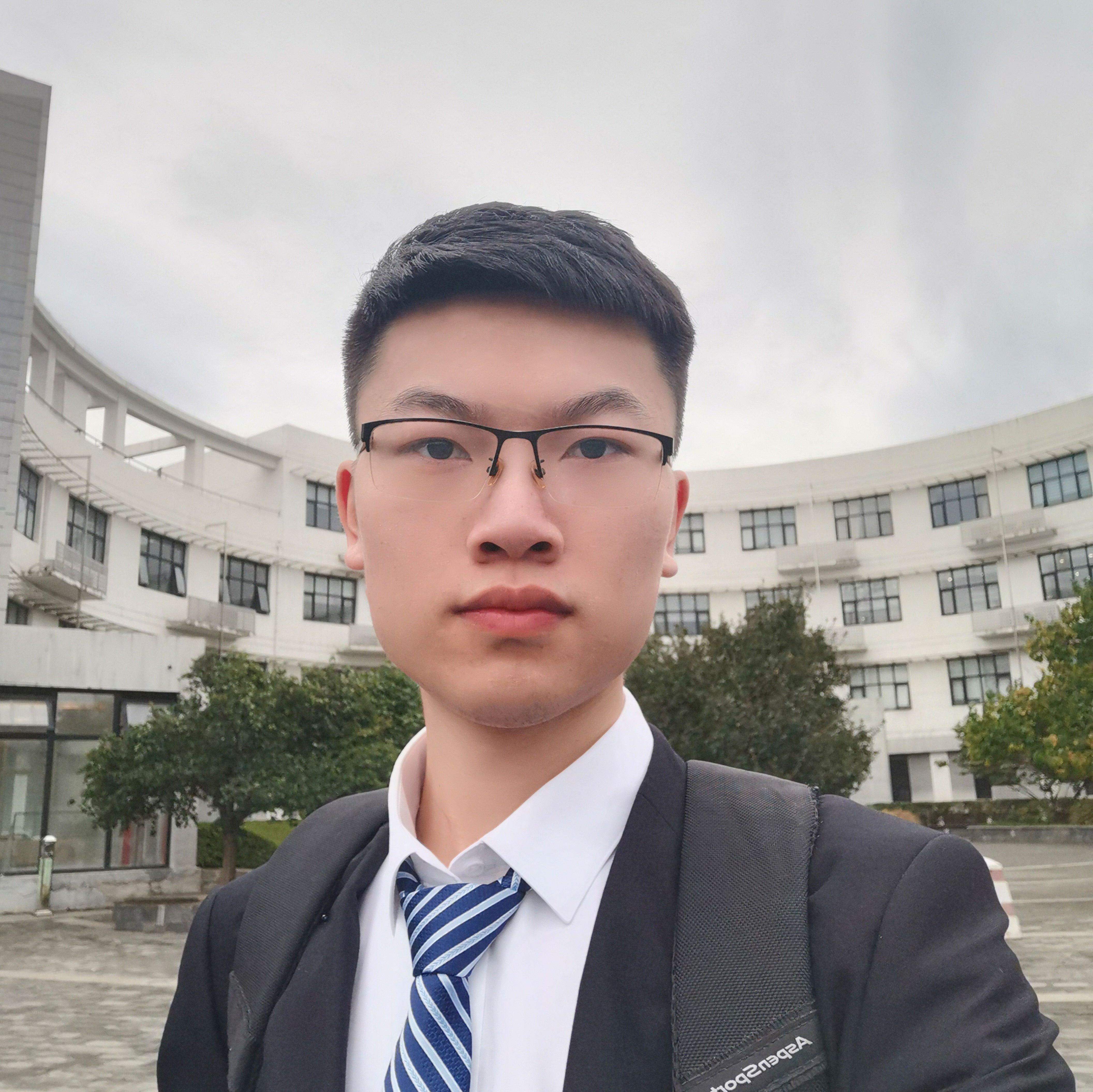
I am a second-year Ph.D. student at Caltech in the CMS department. I’m excited to be a part of the Computational Camera Lab under the guidance of Katie Bouman, and co-advised by Yisong Yue and Yang Song. My research focuses on controlled generation and solving scientific inverse problems with diffusion models.
I earned my B.S. in Computer Science from the Yao Class at Tsinghua University, where I had the privilege of working with Yi Wu and Yang Gao. During a visit to Carnegie Mellon University, I worked with Jun-Yan Zhu, Eli Shechtman, and Richard Zhang.